Thought leadership should be fun, fast, and fearless. You tap into excitement about new ideas and advocate for something better. That’s the mindset behind The Idea Sled. Projects glide forward gracefully. It’s the momentum of commitment. This newsletter shows you how.
It may seem anathema to think of using generative AI to create thought leadership. If writers or marketers did nothing but enter a basic prompt and publish the output as-is, the result would fall far short of what counts as thought leadership. It would neither be thinking or leading.
However, AI also offers much more nuance than that reductive scenario. It is entirely legitimate to use AI to help create thought leadership, even in areas as specialized as institutional finance and capital markets.
Below are seven powerful use cases where professionals (thought leaders or those supporting them) can derive value from AI, based on my recent experiments with several tools.
1. Interview Prep
AI tools can give you a quick assist when preparing to interview a subject matter expert or business leader. Most strategists and writers typically have an initial set of questions and question types. I’ve developed a series of such questions based on commonalities across institutional finance and capital markets topics. They vary based on where my client plays in the value chain or asset lifecycle.
When beginning a less familiar topic, I use a Custom GPT I’ve created in ChatGPT for interview prep. The basic structure is as follows:
I’m interviewing an expert on [topic], and I’m interested in exploring [angles]. Below are my initial questions. Begin this task by asking me to provide the topic and angles. Then help me tailor the questions to this interview. Suggest additional angles and questions as well.
[pasted questions]
My own expert judgment is still needed to identify which angles and questions will best suit my goals. In addition, the output only works as a starting point. It doesn’t replace conducting the interview as a natural conversation and asking good follow-up questions. In fact, it’s entirely normal to cover only about half of the planned questions if you’re a nimble and intellectually empathetic interviewer.
2. Transcription
Using AI for transcripts is a no-brainer. There are many speech-to-text platforms out there. I prefer to use MacWhisper with local models installed to ensure my transcripts don’t become training data.
I’ve also seen good results with the built-in transcription on conferencing platforms. You can change the file extension from .vtt to .txt so that cloud-based models don’t use them (if you don’t have your own running locally).
At this stage, you can also provide AI with the angles and questions you planned to explore and ask it to organize the transcript accordingly.
It’s also a good idea to use AI to clean up transcripts. If you specify proper names and specialized terms of acronyms, AI tools can help clean up their initial output.
3. Additional Research
You can’t cover everything in an interview. I also believe you shouldn’t expect a senior thought leader to educate you on basic details. Many AI tools can help make much more respectful use of interviewees’ time.
For broad research on a topic and the latest trends, I’ve found PerplexityAI best at helping quickly gather some basic orientation and sources for information and data.
The key is not relying on its output at face value. It sometimes makes odd choices for sourcing. It also seems prone to hallucinations. But because it provides sources, it is easy to double-check.
In addition, newer so-called “deep research” features in several AI models can perform much more exhaustive searches with a reasonably useful synthesis of the results. As with Perplexity, however, using these results as-is can lead to embarrassing mistakes. I still use solid research and critical thinking skills to validate what it produces. Perhaps more important, it also takes good judgment to know what’s relevant and what is not.
4. Rapid Synthesis
Working in institutional finance and capital markets means wrestling with often copious and dense materials. You may have to make sense of several technical whitepapers, regulatory documents, government and NGO reports, and more.
Most chat tools do a decent job of summarizing individual documents. However, the best platform for multiple sources is Google’s NotebookLM. It ingests multiple documents, summarizes them individually, and allows you to “chat” with sources by asking questions.
Because NotebookLM is intentionally designed not to be creative in its output, it’s generally less likely to make up facts. For auditory learners, it also has an option to create a podcast-style audio summary of your sources.
5. Outline Validation
I have developed an additional Custom GPT to support the outlining process. It’s generally beneficial for a thought leadership writer to wrestle with shaping a topic into an outline first. Outlining is a great way to plan readers’ trajectory from what they already know and believe to what you want to influence them to think. In my experience, AI doesn’t have a good implicit sense of that shift.
However, once you have an outline, AI can help validate it. The key pieces of my custom prompt are questions about whether the outline is an effective structure for what the thought leadership is meant to accomplish (which is something I specify). About half of what it suggests turns out to be worth considering, with maybe half again of that worth trying to integrate. It’s also a decent proxy for less savvy readers.
Once I have a validated and updated outline, I also ask this GPT to ingest the structured transcript (see above) and begin organizing points from the transcript accordingly. It’s another helpful shortcut, but it often takes a bit of massaging to get everything properly categorized. I’d still say it cuts about half the time it would take to do it manually.
6. Draft Validation
I’ve written for long enough that it’s simply faster and better for me to draft thought leadership pieces myself. A few tests I tried using AI tools to draft turned out to take more time to fix than starting from a blank page. AI output can even be deceptive because it looks and reads enough like human prose that a novice writer might struggle to improve it.
That said, I do get value from using various tools to review drafts. I use a series of canned prompts.
- Read and analyze the attached draft and summarize the main messages.
- Imagine that you are a [intended audience description] who wants to know/do/decide [description]. Does this piece help you with that, and what would make it more valuable?
- Comment on the strengths and weaknesses of this piece, focusing on structure and flow.
- Point out any repetitive phrases or word choices and identify any proofreading issues (Claude excels with this one, but ChatGPT is getting better).
As with other use cases, I take everything with a grain of salt, but typically the process does result in a few notable improvements and surfaces at least a few things I could have overlooked.
A common theme here is that a writer’s discretion is essential to filtering through suggestions.
7. Repurposing
Like it or not (and for the most part, I really don’t), thought leadership will be repurposed into social posts, email copy, ads, SEO blogs, and even translations. Generative AI can do a great job with these mundane tasks.
Generalist platforms, like the major chatbots, do decent work on utility content. However, I’m more partial to dedicated tools. My personal preferences are Spiral and Jasper. Mileage will vary. Getting well-written drafts from them still takes a strong sense of what you’re trying to accomplish, but if you provide that input and prepare to strip out clunky wording and emojis, you’ll take most of the effort out of this low-value task.
For translation, I’ve had the best experience with DeepL, a tool optimized for translation. But I’ve also found that it still takes guidance with technical language. Global financial professionals often use anglicized terms even when local languages have a possible translation. You frequently have to untranslate key vocabulary or adjust to nuances of how people in an industry think. I’ve therefore limited my experiments with AI translation to languages I already know reasonably well.
The Current State of AI (March 2025)
While AI is still not nimble, expert, or psychologically aware enough to produce thought leadership, it can help with many of the more time-consuming elements. ChatGPT seems to be the most useful in general, but specialized solutions can also play a part. I frankly have not spent as much time with CoPilot or Gemini.
But there’s a major caveat. It still takes domain expertise to know what matters and know what to do with it, let alone deliver it in compelling prose that changes what readers know and believe.
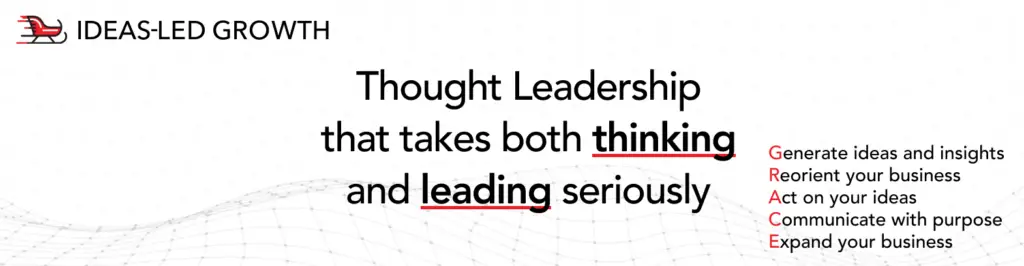
Three Grace Notes
“You have to have your own myth. To have your own myth means to have suffered and struggled with a question until an answer has come to you from the depths of your soul. That does not imply that this is the definitive truth, but rather that this truth which has come is relevant for oneself as one now is, and believing in this truth helps one to feel well.” —Marie-Louise von Franz, Creation Myths
“Beyond that or above all, it was a dense linguistic-literary agglutination, a great edifice of tropes and metaphors” —Guido Morselli, Dissipatio H.G.: The Vanishing
“The trouble is not in the methods themselves, which are excellent in their own sphere. It lies in the sweepingness, the dramatic zing, the naive academic imperialism that insists on exporting them to all sorts of other topics.” —Mary Midgley, The Myths We Live By
Note: The links above are affiliate links. I’m using them in lieu of paid subscription tiers or digital tip jars. Seems like a much more graceful way to generate financial support while sharing more thinking and writing that can guide thought leadership.